Cancer
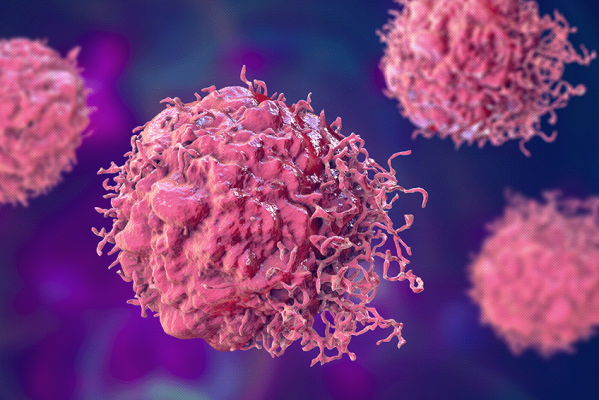
The SciLake Cancer Pilot has been developed to study how to create accessible, interconnected scientific resources within the cancer research community. Led by teams from the Karolinska Institutet (KI) and the Centre for Research and Technology HELLAS (CERTH), this pilot focuses on enhancing the understanding of cancer biology and treatment, specifically targeting Chronic Lymphocytic Leukemia (CLL), the most common form of adult leukaemia.
Innovation in Personalized Medicine
The Cancer Pilot addresses critical challenges in personalised medicine, particularly identifying key biomarkers for tailored treatment approaches. This pilot aims to build a targeted scientific knowledge graph by combining data from biomedical knowledge graphs with new insights extracted through text and graph mining algorithms. The resulting graph will provide a deeper understanding of CLL's heterogeneous nature and other cancers, ultimately improving treatment strategies and patient outcomes.
The CLL Knowledge Graph (CLL-KG)
Our CLL Knowledge Graph brings together vital data from multiple sources:
- Genetic information
- Protein interactions
- Metabolic pathways
- Drug development data
- Clinical trial results
This semantic structure of knowledge graphs is anticipated to be pivotal in enabling researchers and clinicians to gain deeper insights into patient subtypes, treatment responses, and emerging therapies.
Advanced Technology Integration
We're leveraging cutting-edge tools in:
- Text mining and analysis
- Entity recognition
- Graph mining
To accelerate knowledge discovery and advance precision medicine in cancer research.
What we've achieved so far
Our team has made significant progress on several key fronts:
- Developed a comprehensive definition of the Knowledge Space:
- key domain-specific entities: proteins, genes, pathways, diseases, and scientific publications.
- Investigated third-party Knowledge Graphs for integration into our CLL-KG.
- Built and customized a Cancer Research OpenAIRE Gateway to optimise the identification of cancer-specific research outputs.
- Built an extensive article database for testing components and services, and provided feedback on AvantGraph and BIP! Spaces prototypes.
- Developed the first version of the CLL-KG that maps key domain entities, their relationships, and valuable metadata attributes, using a subset of the data.
Related News
- Presentation: Roadmap for a Cancer Knolwledge Graph, presented at the workshop “Open Science Knowledge Graphs: Transforming the Way we Manage, Explore, and Analyze Scientific Knowledge” at Open Science FAIR 2023.
- Workshop: Defining the Roadmap for a European Cancer Data Space, organised by the EOSC4Cancer project in Brussels, October 2023.
- Presentation: Unlocking insights in Cancer Research through Knowledge Graphs at the Cancer Landscale Partnering meeting, February 2024.
- Press release: Connecting the Dots between Cancer Data Networks: The SciLake Cancer Knowledge Graph, April 2024.