SciLake at the Transport Research Arena
SciLake at the Transport Research Arena
SciLake representatives participated in the 2024 Transportation Research Arena, where they presented on the use of Knowledge Graphs in Transport Research. This article recaps the key points from their contribution.
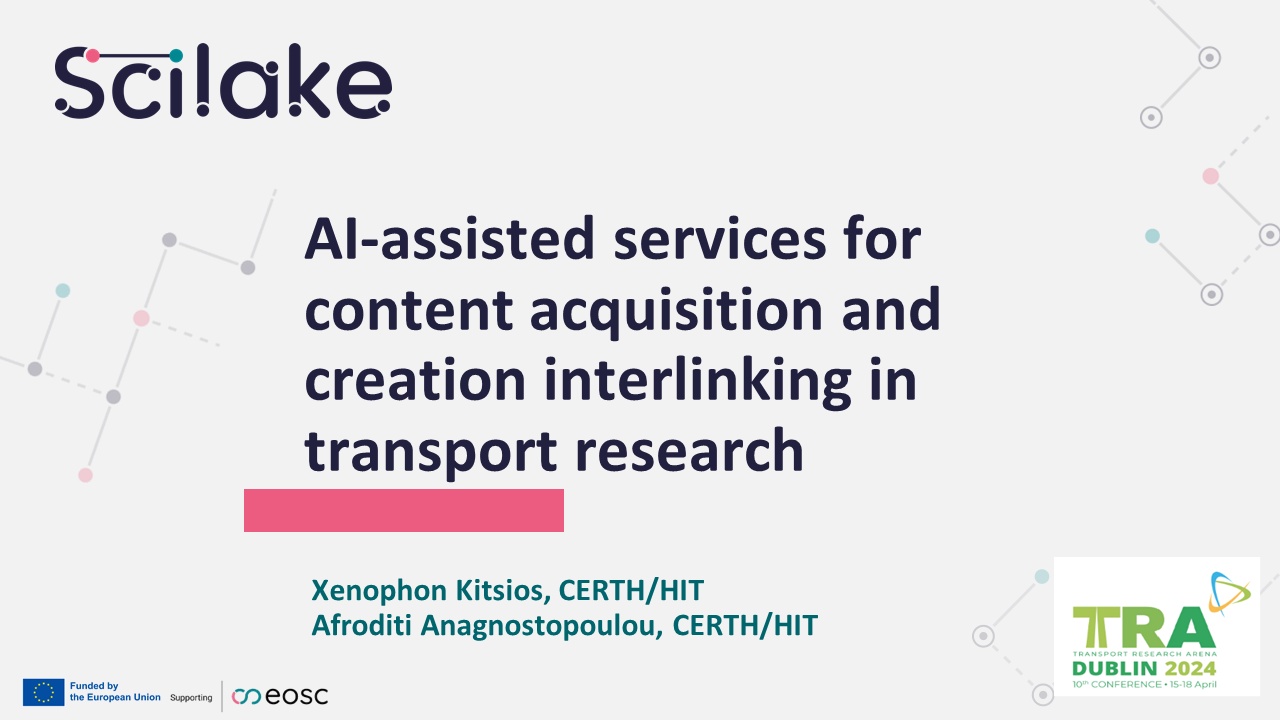
The Transportation Research Arena (TRA) is the largest research and technology conference on transport and mobility in Europe. The 2024 edition took place from April 15 to 18, 2024, in Dublin, Ireland, and focused on "Transport Transitions: Advancing Sustainable and Inclusive Mobility". The conference gave researchers, industry professionals, policymakers, and other stakeholders from all transport modes an opportunity to shape research and transport policy. Key attendees included the European Commission, various European Research Advisory Councils and Technology Platforms, and the European Transport Research Alliance.
Afroditi Anagnostopoulou and Xenophon Kitsios, researchers from the Hellenic Institute of Transport (HIT) at CERTH, showcased the SciLake transport pilot, with a presentation entitled "AI-assisted services for content acquisition and creation interlinking in transport research" in the session "National and Cross-Border Data Interoperability".
Knowledge Graphs in Transport Research
The pilot explores using Knowledge Graphs (KGs) in maritime transport research for:
- Semantic search: Retrieve relevant information and answer complex queries.
- Recommendation systems: Understand user preferences and item attributes.
- Question answering: Extract key information from large knowledge bases to answer user queries.
- Natural language processing: Recognise entities, perform sentiment analyses, and summarise text using semantic context.
Who can benefit?
Content Providers: To automatically verify compliance of exported records with set guidelines
Research Organisations: To support the adoption of Open Science publishing practices and monitor the implementation of Open Science practices by their researchers
Public Authorities: To control the impact of their funding within the Transport research community
What information is included?
- Results representing the outcomes of research activities
- Projects funded by research grants
- Data sources from which graph object metadata is collected
- Communities, or groups of people with a common research intent
- Organizations, including companies, research institutions, and public authorities
Use cases
The pilot has yielded some preliminary results and identified use cases such as:
- 1st scenario: interest of researchers in available software that provides algorithms
- 2nd scenario: use of datasets for optimisation purposes in the transportation sector
- 3rd scenario: publications about “automation” in various transportation domains.
Conclusions & Future Research
In summary, the presentation underscored our commitment to advancing transport research, with a key focus on open-access resources and reproducibility. There is promising ongoing work in SciLake to develop domain-specific Knowledge Graphs (KGs) and AI-assisted services for scientific content acquisition and interlinking.
Currently, two "sub-graphs" are under development: one for the Maritime Transport sector, and another for the Cooperative Connected Automated Mobility (CCAM) sector.
This work has been positively received by representatives of the European Commission and professionals in the maritime transport sector, who see value in the research support and collaboration opportunities that SciLake provides.
Learn more about our pilots: